November 22, 2023
The term “artificial intelligence” (AI) in biotechnology is often nebulous and open to interpretation. Generally, it’s seen as a computer program capable of learning and making predictions from data sets. This broad definition leaves AI’s role in biotechnology somewhat indistinct. For instance, its revolutionary status remains a matter of debate, as exemplified by mixed reactions to DeepMind’s AlphaFold, which accurately predicted the structure of numerous proteins.
AlphaFold’s achievements, while significant, don’t encompass the entirety of AI’s application in biotechnology. AI’s groundwork spans various sectors in biotechnology, with numerous companies integrating it into their workflows. Recognizing AI’s prevalence is key to understanding its role: it’s not an emerging trend but a well-established, diverse tool capable of delivering tangible results. In fact, effective AI in biotechnology could prove invaluable, potentially surpassing the contributions of traditional bench lab scientists.
AI: Function Over Definition
The broad definition of AI – big algorithms processing extensive data – might suffice due to its functionality. The impact of AI systems largely depends on their development and application.
For example, Agilent Technologies has ventured into AI, collaborating with Visiopharm for AI-driven cancer pathology studies. This partnership reflects a blend of diverse methods. Agilent’s Anja Tsalenko, PhD, and Stephen Laderman, PhD, highlight that advanced AI models often involve machine learning and deep neural networks, benefiting from modern computing power, data availability, and advances in related scientific fields.
Meanwhile, Carl Hansen, CEO of AbCellera Biologics, warns against oversimplifying AI as a general category due to its varied definitions and applications in drug discovery, which range from highly effective to superficial.
Offering another viewpoint, Fabrice Chouraqui, PharmD, of Cellarity, sees AI as an enhancement to existing paradigms rather than a standalone solution. He emphasises AI’s reliance on data quality, noting that AI’s effectiveness is limited by the data it processes. This underscores the importance of accurate data in realising AI’s potential in drug discovery and development.
AI in Image Analysis
Biologically significant patterns emerge not just from data sets but also from images of cells, tumours, and tissues. Traditional methods of analysing these images can be labour-intensive and inefficient. AI comes into play here, offering enhanced image analysis capabilities. As noted by Tsalenko and Laderman, AI can transform both fundamental research and medical pathology by enabling the efficient identification of patterns in microscopy images, some of which may be challenging for the human eye to detect.
This technology is revolutionising the digital transformation of microscopy-based tissue analysis in clinical pathology laboratories, potentially improving the accuracy of cancer diagnosis and treatment. In live-cell imaging, AI contributes to tracking the dynamic changes and evolution of cells under study.
Accelerating Drug Discovery and Development
Stacie Calad-Thomson, PhD, Chief Strategy Officer and Head of Drug Discovery at BioSymetrics, sees AI as a key tool in streamlining drug discovery and development. She outlines three primary advantages of AI in this domain: speeding up the discovery of new therapeutics through informed decision-making, enhancing the understanding of biological mechanisms and diseases, and identifying specific patient populations for targeted treatments by connecting phenotypes with genetic and biomarker codes.
Calad-Thomson highlights that while current AI successes focus on chemistry and molecular design for efficiency, the longer-term challenge lies in biology. Successfully integrating diverse patient data with AI from the start of drug discovery is poised to become the standard approach.
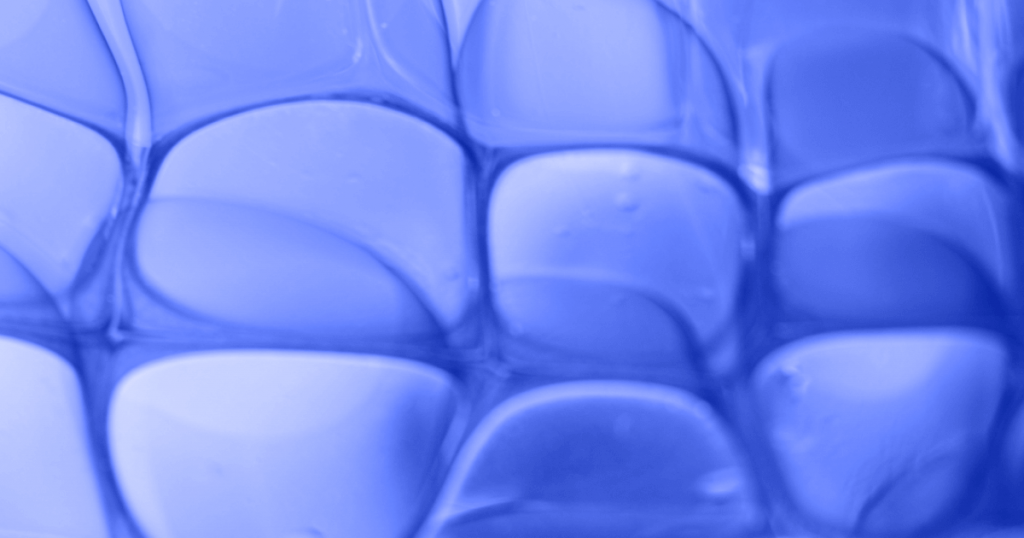
The Interplay of Data and Drug Development
Chouraqui emphasises that our understanding of disease, traditionally limited to malfunctioning cells and organs, has been too narrow, leading to a reductionist approach in treatment development. Focusing solely on single molecular targets often fails to address the complexities of biology. He asserts that as the drug development process advances to higher species, initial assumptions may not hold, underlining the need for a more comprehensive approach that AI can facilitate.
Integrating Data Quality in Cell-Level Drug Development
As Cellarity exemplifies, shifting from a target-centric to a cell-level approach in drug development requires the collection and analysis of vast amounts of data, where quality is paramount. The relationship between high-quality data and superior analytical work is crucial in this context. When Chouraqui was asked about the diminishing role of experimental biology in the era of algorithm-driven drug discovery, he disagreed, emphasising the continuous need for a blend of wet and dry lab work. He explained, “It’s an ongoing loop. Access to and generation of high-quality assets are vital. You need to evaluate the AI’s output and reincorporate those findings into the AI process.”
AbCellera’s Hansen concurred, stating, “The companies that will most effectively leverage AI are those with robust experimental capabilities as well.”
Unravelling the Complexities of AI in Drug Development
While AlphaFold’s breakthrough in protein folding initially seemed like it could revolutionise molecular interaction studies, Hansen points out that simply knowing a protein’s structure doesn’t necessarily expedite drug discovery. He noted, “We’ve accumulated over 65,000 structures in the Protein Data Bank, but there’s more to drug discovery than just understanding protein structures. The journey from recognising a protein structure to defining a potential drug involves many intricate steps.”
This perspective underscores that while AI and data-driven approaches are transformative, they are just pieces of a larger puzzle in drug development. The journey from data to drug discovery is complex and multifaceted, necessitating a comprehensive approach that blends experimental biology with advanced computational methods.
Our expert team specialises in identifying top talent in AI and Biotech, ensuring your organisation stays at the forefront of technological advancement. If you’re seeking to enhance your team with key players who can drive AI innovation in the biotech sector, we invite you to visit our website for more details about our executive search services. Should you wish to discuss your particular requirements, please don’t hesitate to reach out to us at a time that suits you.
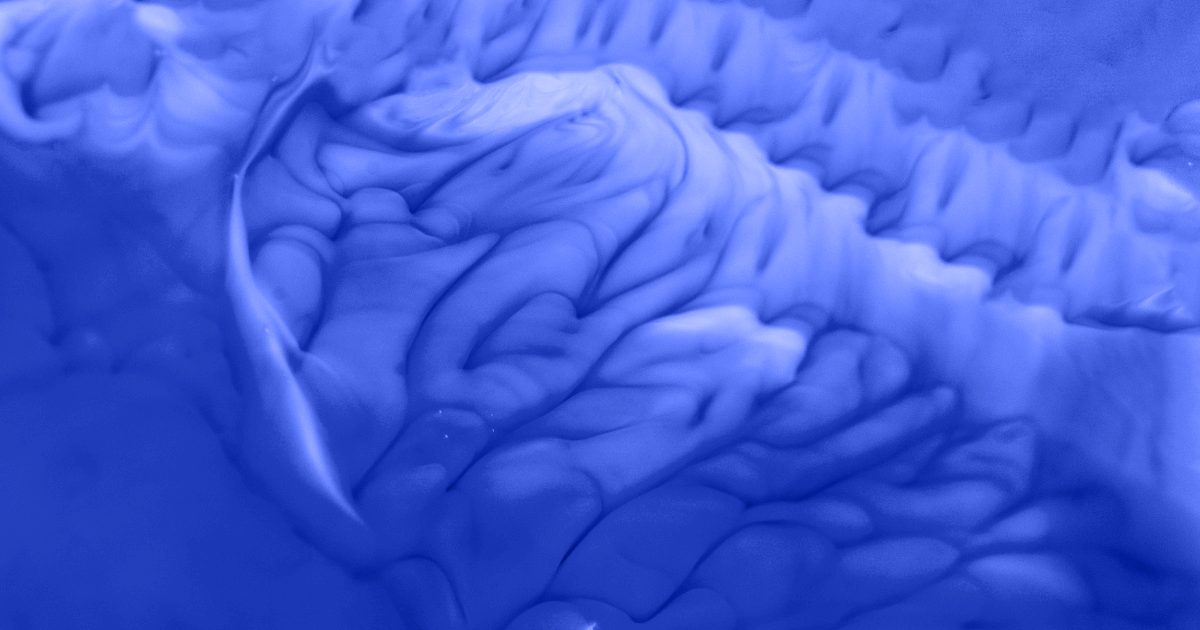
Published on 22-11-2023